Novel Algorithms Merge Ground- and Space-based Data to Forecast Air Pollution Events
05/03/21 – Air pollution kills approximately seven million people each year, according to the World Health Organization (WHO). That’s a life lost every five seconds to a variety of preventable cancers, infections, and other illnesses afflicting communities struggling to maintain clean air.
“Those deaths are tragic. Cities use lots of different strategies to try and protect people, but our mitigation strategies are limited by the amount of air quality data we have at our disposal,” said Jeanne Holm, Deputy Mayor of Budget and Innovation for the City of Los Angeles.
Sunrise over the City of Los Angeles. Researchers in LA are developing a new tool cities can use to protect their populations from air pollution. Image credit: Martin Adams
With a grant from NASA’s Earth Science Technology Office (ESTO), Holm and a team of researchers are working on advanced machine-learning software that could make it easier for cities to forecast air pollution events. Their project, aptly titled “Predicting What We Breathe (PWWB),” combines deep neural network models with other classic machine-learning algorithms to identify relationships between air quality data gathered from ground sensors in Los Angeles and imaging data gathered from Earth-monitoring satellites managed by NASA and the U.S. Geological Survey (USGS). Understanding these relationships would enable scientists to train systems used to process data from sources like Terra MODIS, Aqua MODIS, and Landsats to detect signs of impending air pollution incidents too subtle to be detected by in-situ sensors in LA In addition, PWWB would help researchers not only better classify urban air patterns in LA, but also allow them to detect similarities between those air patterns and other air regimes in megacities around the world. This capability would enable urban communities worldwide to protect their citizens from the harmful effects of air pollution with data-driven mitigation strategies.
“Our goal is to create a tool anyone can use to predict and prevent air pollution events. We want to empower other cities to keep their citizens safe as well,” said Holm.
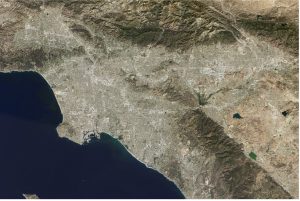
Landsat image of Los Angeles, captured by the Operational Land Imager (OLI) instrument on Landsat 8. With PWWB, Landsats would be able to locate signs of an imminent air pollution event in images like this, enabling cities like LA to forecast air pollution events with greater accuracy. Image credit: Jesse Allen, using Landsat data from USGS
Los Angeles already uses an extensive network of air quality sensors to keep tabs on pollution. These sensors are in LA’s ports, parks, and even streetlights, providing officials with critical information about the air their citizens breathe. If air quality is poor, the city can issue an advisory encouraging citizens to take precautions.
“Effective forecasting is one of the best ways to keep citizens healthy. It gives people who are particularly prone to respiratory illness time to connect with their healthcare provider and start preventative treatments. Being proactive is key to avoiding a health emergency,” said Dawn Comer, LA’s Broadband and Digital Inclusion Coordinator.
But LA’s system for detecting air pollution is limited to the Los Angeles metropolitan area, and events far away from the city can have just as big an impact on air quality as traffic on Ventura Boulevard. For example, wildfires in other parts of California could jettison large amounts of particulate pollutants into LA’s air and cause an unexpected spike in air pollution.
“Earth is a system of systems. If we want to effectively mitigate air pollution, we need to be able to look at the big picture and understand how our city fits within the broader environmental picture,” said Holm.
To discover relationships between data gathered from ground-based air quality sensors and NASA satellite observations, PWWB will use multimodal deep learning algorithms. These algorithms include several deep Recurrent Neural Network (RNN) models, which are ideal for forecasting outcomes from time-series data. That makes them well-suited to comb a wide variety of data sources—including sensor measurements, satellite images, meteorological data, and wildfire data—to locate patters between information from LA’s sensor network and images collected by NASA and USGS. Essentially, these models are teaching data classifiers to identify signs of an impending air pollution event in the images of Earth’s surface that satellites already gathered of Earth’s surface.
“Any relationship between our air quality data and Landsat images could be too nuanced for human analysts to detect, so we need to develop new information systems to find these patterns for us,” said Holm.
Perhaps the most remarkable aspect of PWWB is its flexibility. Holm, who served the Obama Administration as an appointed Open Data Evangelist, says that her team will release their modeling software open-source on the popular coding site GitHub once it’s complete, allowing anyone with a computer to access their work and tailor it to fit any urban environment in the world.
“Air pollution isn’t just an LA problem. There are people all over the world who are struggling to stay healthy in polluted urban environments, and if others can use PWWB to mitigate air pollution more efficiently, then it can only help us all in the long run,” said Holm.
Preparing PWWB for the general public is no small feat. Holm credits her team, especially her Co-Investigators at California State University, Los Angeles, and the non-profit OpenAQ, for making this project possible.
“Dr. Mohammed Pourhomayoun’s team at California State University, Los Angeles, is instrumental for unraveling the complexities of normalizing satellite and ground data with varying scales of time and space, and Jeremy Taub’s team at OpenAQ unites collaborators from the LA area with cities around the globe to help us apply these algorithms to real data sets collected from each of their cities,” said Holm.
Holm also credits ESTO for helping her team transform their technology concept into a world-ready tool. In particular, scientists with ESTO’s Advanced Information Systems Technology (AIST) program were crucial for providing Holm’s team with the funding and expertise they needed to turn their vision into a reality.
“PWWB brought together a lot of brilliant, hard-working people, and ESTO equipped those people with the tools they need to succeed. We have to work together to create novel technologies for studying Earth systems,” said Holm.
PWWB is still in the early phases of development, but Holm hopes to have a valid prototype algorithm prepared by July 2021.
“There’s a lot of work to be done, but using machine-learning to process data from space-based remote sensors can really change the way we forecast air pollution events,” said Holm.
Gage Taylor, NASA Earth Science Technology Office