Automated metadata pipeline may make NASA data more accessible to Earth scientists
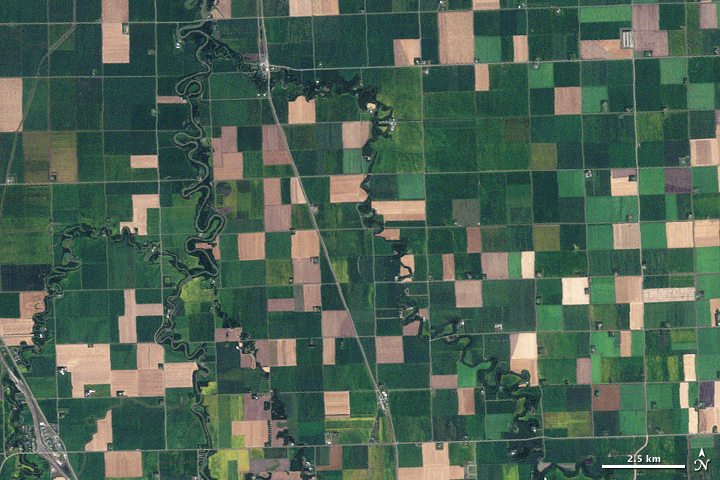
A Landsat image of farmland in Minnesota. AMP metadata records could be used to accelerate research on the complex relationships between land use and other Earth system phenomena that affect human life. (Credit: NASA Earth Observatory)
If you’ve ever struggled to find information using an online search engine, then you know how difficult research can be. This is especially true for professional scientists, who must search through troves of data to construct their models and hypotheses.
“Finding ideal data isn’t always as easy as submitting a query through a search bar. Many data sets simply aren’t organized in a way that makes them visible to search algorithms,” said Beth Huffer, founder and CEO of Lingua Logica, LLC. “And finding the data is only the beginning. Making it ready-to-use can be even more challenging.”
With a grant from NASA’s Earth Science Technology Office (ESTO) Advanced Information Systems Technology (AIST) Program, Huffer wants to help researchers locate, access, and use NASA Earth science data sets with greater ease. Her project, Automated Metadata Pipeline (AMP), would automate the process of annotating NASA data sets with descriptions of what, where, when, and how the Earth science phenomena represented in the data set were measured. That information – also known as metadata – would then make it easier for search engines specializing in scientific discovery to connect researchers with data sets most relevant to their research goals and enable software developers to create Application Programming Interfaces (APIs) that connect Earth science data sets with data analysis applications. This would lead to improved models describing everything from climate change to agricultural productivity.
“NASA gathers petabytes of data each day. If we don’t have an efficient process for turning that raw data into data products for scientists and decision makers, then we aren’t capitalizing on the full value of that information,” she added.
Robust, high-quality metadata is critical for accelerating scientific research, Huffer explains. When we search for something online, it’s the context clues expressed as metadata that allow search algorithms to separate information relevant to a query from information that’s irrelevant. The more descriptive metadata is, the easier it is for those algorithms to generate helpful results. High-quality metadata can even help researchers assemble complex models.
But manually curating metadata for NASA’s Earth science data sets is an onerous and time-consuming task. There are more than 8000 collections in the NASA Earth Observing System Data and Information System (EOSDIS) archives, and each collection can contain hundreds of individual datasets. While tools exist to assist in the metadata process, they generally rely on metadata curators manually filling in forms using drop down lists. Different curators may categorize the same information in different ways, which makes consistency hard to achieve.
“It’s a common complaint among scientists that they spend more time preparing data for analysis than they spend actually analyzing data. Manual metadata curation tends to yield metadata records that use disparate terms and formats, which make it difficult to programmatically prepare and use the data with applications, even when the metadata is very descriptive,” said Huffer.
AMP could help solve this problem. Huffer is working with colleagues at the Basque Centre for Climate Change (BCCC) to provide data for BCCC’s ARtificial Intelligence for Ecosystems Services (ARIES) platform, a network of eco-services models. By teaching convolutional neural nets (CNNs) to organize information according to detailed ontologies, Huffer developed an AMP prototype that automatically produces metadata for the NASA data sets ARIES uses to programmatically identify data that can serve as inputs for models within the ARIES network and satisfy user requests in real time.
“For the prototype, we manually trained a convolutional neural network to recognize about 49 different variables. The neural network was then able to recognize those variables when they occurred in other data sets, and instruct the AMP data annotation module to assign the same labels to the new data sets as those that were assigned manually to the training data. So now we know that it is possible to use machine learning to generate good metadata automatically,” said Huffer.
A tool that automatically generates consistent, semantically-grounded metadata for NASA Earth science data sets would be an immense boon to NASA science, improving the interoperability of NASA’s petabytes of disparate data products and increasing the pace of scientific discovery. Indeed, making data FAIR (findable, accessible, interoperable, and reusable) is one of NASA’s top objectives.
“Something like AMP might ultimately save both metadata curators and researchers hundreds of hours of work,” said Huffer.
For an Earth scientist who works at the intersection of science and technology – such as Annie Burgess, Lab Director at Earth Science Information Partners (ESIP) – the potential benefits are considerable.
“It’s very exciting. AMP has the potential to impact the entire data lifecycle. By streamlining metadata generation, AMP takes a significant burden off of data professionals and researchers, ultimately streamlining the timeline from data generation to scientific insight,” said Annie.
Huffer stresses that there’s still a lot of work to be done, but her team’s recent success with the AMP prototype is promising. She wants to continue working with NASA to develop her technology concept further and, ultimately, share AMP with metadata curators at NASA’s Distributed Active Archive Centers (DAACs), who help catalogue and maintain NASA’s collected Earth science data. She is also eager to explore other uses for the AMP data preparation pipeline.
“AMP will not only reduce the cost and the amount of time it takes to produce robust, highly descriptive metadata records, but will also ensure that the language and format used in the descriptions are consistent,” said Huffer.
ESTO’s AIST program identifies, develops, and supports novel software and information systems like Huffer’s technology. For more information, please visit AIST’s webpage.
Gage Taylor, NASA Earth Science Technology Office